Parameter analysis of texture feature in oil spill detection based on SAR
-
摘要: 溢油已是当前海洋生态环境破坏的主要因素之一,因此对海洋溢油的检测分析是当前海洋环境保护的一个重要课题。传统的溢油提取仅仅是单独依靠光学影像的光谱信息或者合成孔径雷达(SAR)影像的后向散射系数信息进行提取,这会造成很多同谱异物或者粗糙度相近似的地物错分,因此除了利用传统的影像信息以外,还需结合影像的纹理信息,从而提高溢油提取的精度,减少错分地物的数量。选用2006年渤海地区的三景同轨SAR影像作为数据基础,应用基于灰度共生矩阵的方法对其进行纹理分析。该方法可以很好地对图像区域和表面进行感知并能够从像元的灰度相关性上对纹理特征进行详细描述,因此适合于SAR影像的海洋溢油检测。在纹理分析的过程中有很多的参数需要选择,参数选择的好坏将直接影响最终提取结果的精度。通过对纹理分析过程中的参数进行讨论、实验、选择与验证,最终确定了基于灰度共生矩阵纹理分析中各参数的值,并选择了局部平稳、非相似性、对比度、变化量4个特征量作为溢油提取的纹理特征统计量。将纹理特征与SAR自身的后向散射系数相结合,通过神经网络分类法对其进行分类,并计算出分类精度为80.65%,分类效果良好。由此说明了将影像的传统信息与纹理信息相结合进行溢油提取是一种可行而有效的方法,同时也为后续的海洋溢油检测工作奠定了一定的基础。Abstract: Oil spill in ocean has been one of the primary factors which lead to deteriorations of marine ecological balance. The analysis of marine oil spill detection has become an important subject of the marine environmental protection. However, traditional methods to extract oil spill merely base on the spectral information of optical image or backscattering coefficient of SAR image respectively, which may lead to misclassification of different objects with same spectrum or similar roughness. Hence, texture information is combineal with the traditional image information to improve the extraction accuracy of oil spill. In the process of texture analysis, there are many parameters which will directly affect the extraction accuracy. So it is important to select appropriate parameters. In this paper, we choose three SAR images in the same orbit which covered the Bohai Sea area in 2006 as data resource, and use method based on gray level co-occurrence matrix (GLCM) to analyze texture feature. Because GLCM-based texture analysis method can perceive the surface of image well and describe the texture feature in detail by gray correlation of pixels, it is more suitable for marine oil spill detection in SAR images. Then we discuss, experiment, select and verify the parameters of texture analysis. Finally, this paper selected four parameters include local stationary, non-similarity, contrast and change as the texture feature statistics, determined the value of these parameters, used neural network classification considered both texture feature and backscattering coefficient of SAR. With the classification accuracy up to 80.65%, the method combined the traditional information with the texture information to extract oil spill turn out to be feasible and effective and also laid a good foundation for the future study on marine oil spill detection.
-
Key words:
- ocean /
- oil spill /
- GLCM /
- SAR /
- texture feature /
- backscattering coefficient /
- neural network
-
邹亚荣, 林明森, 马滕波,等. 基于GLCM的SAR溢油纹理特征分析[J]. 海洋通报, 2010,29(4):455-458. Mansor S B, Assilzade H H, Ibrahim H M. Oil spill detection and monitoring from satellite image [EB/OL]www. gisdevelop ment.net/appli2cation/ miscellaneous. Topouzelis K,Karathanassi V, Parlakis D.Oil Spill Detection Using RBF Neural Network And SAR Data [C]. 20th ISPRS Congress, Istanbul, Turkey, 12-23 July 2004. Ban Yifang,Hu Hongtao,Rangel I M, et al. Fusion of Quickbird MS and RADARSAT SAR data for urban land-cover mapping: object-based and knowledge-based approach[J]. International Journal of Remote Sensing, 2010, 31(6): 1391-1410. Baraldi A,Parmiggiani F.Classification of sea-ice types in SAR imagery[J]. Nuovo Cimento-Societa Italiana DI FISICA SEZIONE C, 2001, 24: 113-122. 李栖筠, 高超, 付玉慧. 卫星溢油信息提取及应用研究 [G]//第十四届全国遥感技术学术交流会. 中国青岛,2003-10. 胡伏原, 张艳宁, 薛笑容,等.基于树型小波和灰度共生矩阵的SAR图像分类[J]. 系统工程与电子技术,2003, 25(10): 1286-1288. http://finance.ifeng.com/hua.bao/hbyw/20090828/1165831.shtml.[EB/OL] 梁小祎, 张杰, 孟俊敏. 溢油SAR图像分类中的纹理特征选择[J]. 海洋科学进展, 2007, 25(3): 346-354. 赵凌君, 秦玉亮, 高贵,等.利用GLCM纹理分析的高分辨率SAR图像建筑区检测[J]. 遥感学报, 2009, 13(3): 483-490. 胡召玲, 郭达志, 盛业华. 基于小波分解的星载SAR图像纹理信息提取[J]. 遥感学报,2001, 5(6): 423-427. Li W,Benie G B,He D-C, et al. Classification of SAR image using morphological texture features[J]. International Journal of Remote Sensing, 1998, 19(17): 3399-3410. 朱立松. 应用纹理分析和神经网络方法分类海上SAR溢油图像[D]. 大连:大连海事大学, 2005. Wikipedia. Artificial Neural Networks [EB/OL]. http://zh.wikipedia.org/wiki/%E4%BA%BA%E5%B7%A5%E7%A5%9E%E7%BB%8F%E7%BD%91%E7%BB%9C. -
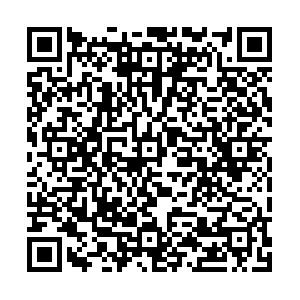
计量
- 文章访问数: 1922
- HTML全文浏览量: 5
- PDF下载量: 1241
- 被引次数: 0